Expanding the Use and Scope of AI Diffusion Models
Story by:
Published Date
Article Content
Researchers at the University of California San Diego and other institutions are working on a way to make a type of artificial intelligence (AI) called diffusion models — a type of AI that can generate new content such as images and videos by training on large datasets — more efficient and widely applicable.
Currently, diffusion models work by making small, incremental changes to input data, allowing the model to learn complex patterns and relationships — a process that can be slow and limited in application. So Yian Ma, an assistant professor at UC San Diego’s Halıcıoğlu Data Science Institute (HDSI), part of the School of Computing, Information and Data Sciences, and his research colleagues have developed a new approach that allows for larger jumps in between steps, making the process faster and more flexible.
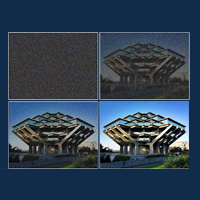
In a recent paper titled Reverse Transition Kernel: A Flexible Framework to Accelerate Diffusion Inference, Ma and researchers at the University of Illinois Urbana-Champaign (UIUC), the Hong Kong University of Science and Technology (HKUST), the University of Hong Kong (HKU) and Salesforce AI Research presented an analysis of a generalized version of diffusion models. The paper was recognized as a spotlight paper at NeurIPS — one of the largest conferences in machine learning — and it was awarded best paper at the International Conference on Machine Learning (ICML) workshop: “Structured probabilistic inference and generative modeling.”
“Classical diffusion models incrementally add small, Gaussian noise (a normal random variable with a small amplitude) to transform the data distribution toward a simple, standard normal distribution. The models then learn functions to specify the incremental changes and ‘denoise’ to transform the standard normal random variable back to one that follows the data distribution,” Ma said.
According to Ma, however, the research team does not require the incremental updates to be small Gaussian noise. Instead, they consider larger jumps in between steps that follow distributions beyond the normal ones. These can be long-tailed distributions or even distributions generated by subroutine algorithms. Using this technique, the researchers were able to reduce the number of intermediary steps and accelerate the algorithm for the diffusion models, making them more widely applicable to various tasks.
“We can see that such generalization improves the efficiency of the diffusion models. Potentially, it could also lead to much wider usage of diffusion models, such as language generation and more interestingly long-term reasoning and decision making,” Ma said.
In addition to Ma, the research team includes Xupeng Huang, currently a visiting student at HDSI; Tong Zhang, from UIUC; Difan Zou and Yi Zhang from HKU; and Hanze Dong from Salesforce.
“What's most exciting about this work is that it can make use of almost any intermediary transition step, that can both accelerate the algorithm and make the algorithm more widely applicable to various downstream tasks,” Ma said. “I would expect this work to be applied to text generation and multi-modal generation, long-term reasoning, tool using and problem solving, as well as decision making tasks to both accelerate and improve the outcomes of such tasks.”
Learn more about research and education at UC San Diego in: Artificial Intelligence
Share This:
Stay in the Know
Keep up with all the latest from UC San Diego. Subscribe to the newsletter today.