Smartly Done
Artificial intelligence is on its way to transforming how we understand and treat disease.
Published Date
Article Content
When a stem cell transplant patient arrived at UC San Diego Health with sudden chest pain and difficulty breathing, doctors raced to find a diagnosis. A CT scan revealed pockets of air surrounding his heart and filling the space between his lungs, but it required analysis from a radiologist to connect the dots and solve the medical mystery.
Albert Hsiao, MD, PhD, reviews hundreds of clinical images a day — CT scans, X-rays, MRIs — using his keenly trained eye to identify signs of disease. But unlike standard radiologists, Hsiao is also equipped with an additional tool: artificial intelligence (AI).
Many have hinted at AI's great potential to enhance practices across research and medicine, with applications spanning everything from radiology and surgery to disease modeling and drug discovery, and even patient safety and cybersecurity. But physicians and scientists are still working to translate that potential into real-world use, with UC San Diego faculty among those leading the charge.
As an associate professor of Radiology at UC San Diego School of Medicine and associate director of the Center for Translational Imaging and Precision Medicine at UC San Diego Health, Hsiao leads a prolific research team that specializes in developing AI tools. Their sophisticated software programs augment analyses of all sorts of clinical images.
On this day, he happened to be equipped with a new algorithm for measuring air trapping in the lungs. Such air trapping is invisible to most radiologists, so when the patient received a routine CT scan two months prior, the images appeared normal. But when Hsiao reanalyzed them with this new tool, the AI was able to see something physicians could not.
The machine-learning algorithm was trained to calculate the amount of air trapping in the lungs and highlight any signs of it in the scan in blue. In the dimly lit radiology room, two almost entirely blue lungs appeared on Hsiao’s screen.
“It was the most air trapping I’d ever seen,” said Hsiao.
“An expert radiologist spends years and years reviewing clinical images, training their brain to identify these subtle visual signs of disease. An artificial neural network can learn them in hours.”
The patient had developed spontaneous pneumomediastinum, a rare side effect of his immune response to the stem cell transplant. So much air had been trapped in his lungs that it eventually had to escape into his chest cavity, producing the symptoms that brought him back to the hospital.
The patient’s condition was successfully treated, the utility of the AI algorithm clearly shown.
“If we hadn’t gone back and looked at the lungs this way, we’d have no explanation for how this happened,” said Hsiao. UC San Diego Health now uses this technology to screen for air trapping in stem cell transplant patients.
This is one example of the vast array of AI tools Hsiao and his colleagues have been working on. In each project, the researchers feed clinical scans into a machine and give it a specific task to do, such as calculating air trapping. Using deep learning methods, an AI algorithm reviews the images, looking for any structures and patterns in the data that it can use to complete the task.
“An expert radiologist spends years and years reviewing clinical images, training their brain to identify these subtle visual signs of disease. An artificial neural network can learn them in hours.”
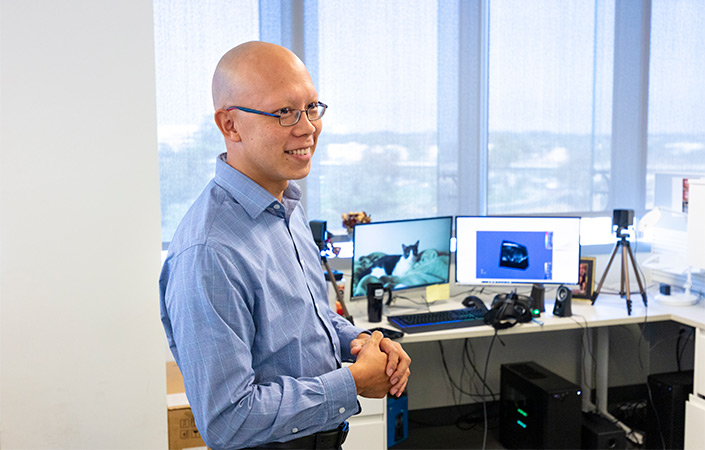
Hsiao’s team has developed tools to detect early signs of pneumonia in X-rays, which became critical to UC San Diego Health’s triage of COVID-19 patients. In another study, they had a machine review thousands of images of heart disease patients, looking to see if there were any common patterns in their cardiac muscle motion. The algorithm identified three distinct groups in the population: one with typical muscle motion, another with a poorly contracting left ventricle and a third requiring pulmonary valve replacement.
"These are patterns that can only be found through painstaking, systematic review of a huge number of clinical scans,” said Hsiao. “Even the best physicians seeing lots of patients aren’t going to be able to spot these things on their own.”
But Hsiao isn’t worried about machines taking his job anytime soon. He sees this collection of algorithms as “another pair of eyes” helping him do his job better and more efficiently.
“A radiologist should be a detective,” he said. “If you’re in a rush to read 400 X-rays just to say if something appears in the image or not, you haven’t actually solved the problem of why the patient is coming for help. If I can get a computer to read those X-rays for me, I could spend that time focusing on patients that really need my attention, or building the next AI tool.”
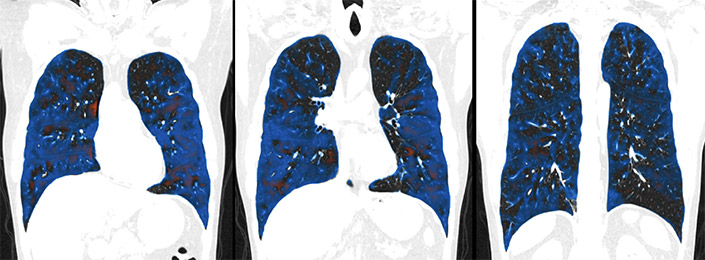
Bridging bench and bedside
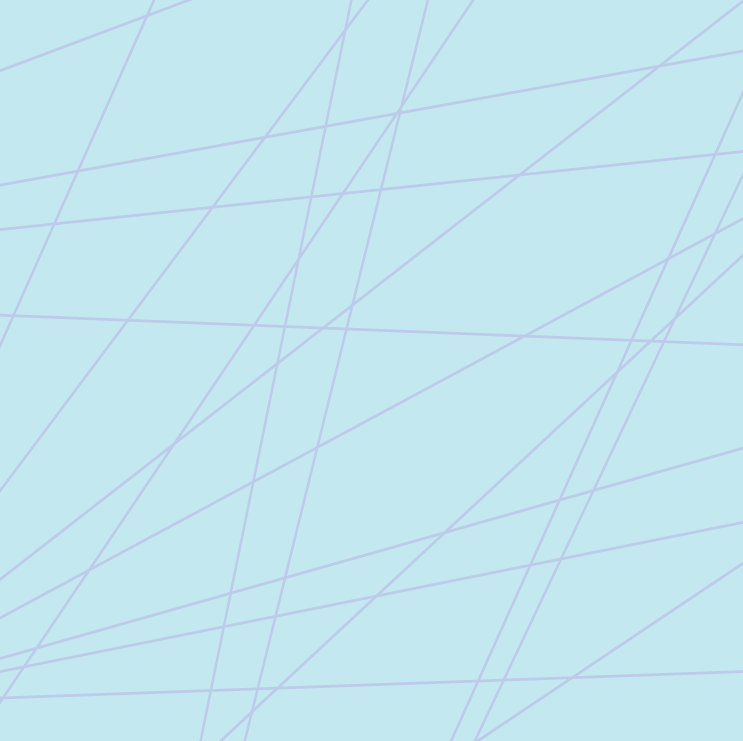
Another application of AI tools is taking off not in the clinic, but in the lab.
Pradipta Ghosh, MD, professor of cellular and molecular medicine at UC San Diego School of Medicine, is one of several researchers advancing the use of AI to develop better models of disease.
Over her years of clinical practice, Ghosh became disillusioned with how little the field could truly explain the origins of many diseases, and how difficult that made it to develop effective treatments.
The classic way to define a disease is through its symptoms. Based on observations in the clinic, the physician’s mind does exactly what an algorithm would do: it looks for patterns and tries to identify the clearest difference between a sick person and a healthy one.
The problem, Ghosh said, is that these overt symptoms usually reflect later stages of disease.
“When we try to define a disease or develop cures for it based on overt symptoms, we often fail, and spend a lot of time and money in the process,” said Ghosh. “In many cases, the most obvious symptoms are red herrings that distract us from the real issue, which is often many biological steps earlier.”
Examples include the significance of amyloid plaques in the progression of Alzheimer’s disease or the role of inflammation and fibrosis in inflammatory bowel disease. Many clinical trials testing drugs that target these symptoms have not been successful, disappointing physicians and patients in the process.
Fortunately, scientists now have much more data to learn from than even just a few years ago and better learning machines. Ghosh’s team is looking to trace disease not through clinical symptoms alone, but through gene expression patterns and the cellular processes to which they correspond.
To do this, they first measure levels of gene expression in models of health and disease, which can take the form of patient tissue samples, human cell lines or lab-grown organoids. They then use AI algorithms to identify differences between them.
“These algorithms are showing us things we’ve overlooked for years and truly changing the way we practice medicine.”
To develop these tools, Ghosh has teamed up with Debashis Sahoo, PhD, associate professor in the departments of Computer Science, Engineering and Pediatrics at UC San Diego. Though trained in different fields, Ghosh said she and Sahoo share a similar philosophy on the cell.
“A lot of complex biology can be boiled down to a few fundamental processes,” she said.
Ghosh and Sahoo see cells as little machines, constantly integrating a slew of input signals and coming up with a molecular response. “But there are really only a few core responses a cell can choose from: to divide or not divide, to live or to die, and so on.”
In their view, each disease comes down to some moment when the cells reach one of these molecular checkpoints and, for some reason, make the “wrong” decision. This event then leads to a cascade of downstream effects that eventually manifests as disease.
“Our goal is to develop algorithms that can look at the data and identify these critical checkpoints along the progression of a disease, and find which genes or proteins are involved at each one,” said Ghosh.
By doing this, researchers will be able to identify what stage of disease a patient is at based on their current gene expression patterns, and suggest a drug that targets the genes most influential at that stage.
We want to catch this domino effect as early as we can in each person, but this is not something a doctor could ever do without AI,” Ghosh said.
The team has already used this approach to advance understanding of numerous diseases, including inflammatory bowel disease, colorectal cancer, Kawasaki disease and COVID-19. In several proof-of-concept projects, researchers have successfully used algorithms to retroactively predict patients’ diagnosis, prognosis and which treatments would work best.
In the case of inflammatory bowel disease, for example, the team was able to map out a network of genes involved in the disease, not only identifying which genes they were, but also grouping them into clusters to reveal which cellular process each group affects. They then used machine-learning approaches to ask which part of this network was contributing most to the disease.
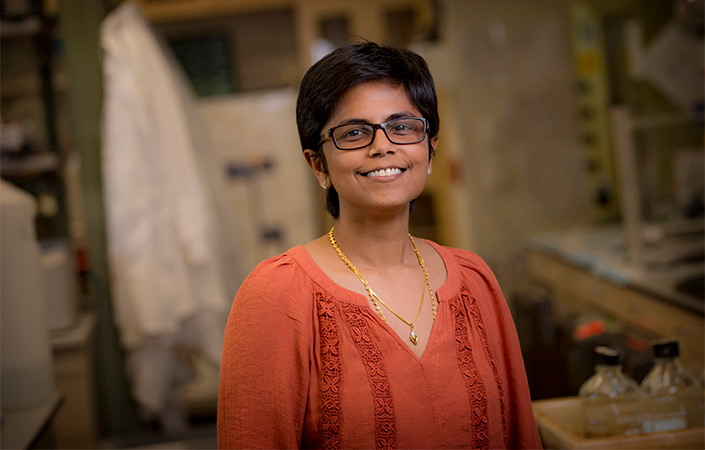
“The answer surprised us,” said Ghosh. “Everyone was focused on the inflammation, but our algorithm said the most important thing was actually the integrity of the epithelial barrier lining the intestines.”
The researchers then identified several gene targets critical to this part of the network. “No one had considered these genes before, but when we reviewed previous clinical trials, the drugs that happen to affect this part of the biology were always the most successful.”
Multiple studies had even documented that the return of tight junctions in the epithelial barrier was the only true determinant of disease remission.
“These algorithms are showing us things we’ve overlooked for years, and truly changing the way we practice medicine.”
Ghosh attributes much of their success to Sahoo’s unique approach to AI. “What sets us apart is the underlying mathematical framework,” she said. “Debashis has pioneered a new way of analyzing gene expression data that is optimized to find these fundamental features of health and disease.”
Ghosh is so invested in this AI strategy that she’s actually left her clinical practice to further focus on this work.
“It’s just really hard to be in the clinic talking to a patient and describing all the things we know about their disease, but still having to say, ‘I’m sorry, I don’t have a drug for you today,’” Ghosh said. “I’m drawn to this work because it feels like a better way for me to have a true impact on these patients’ lives.”
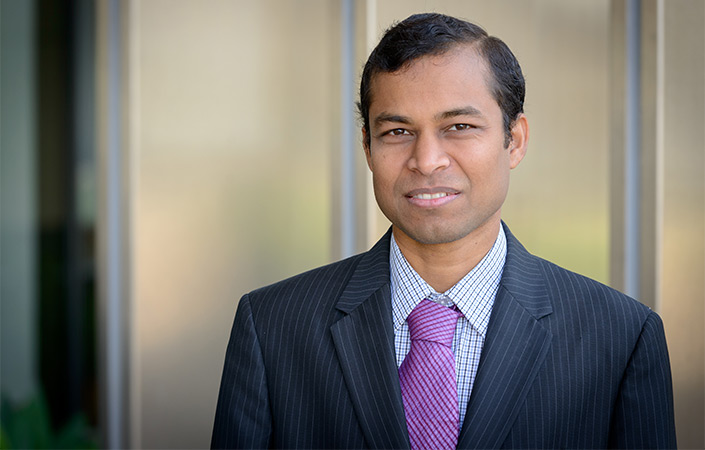
Shining light on the black box
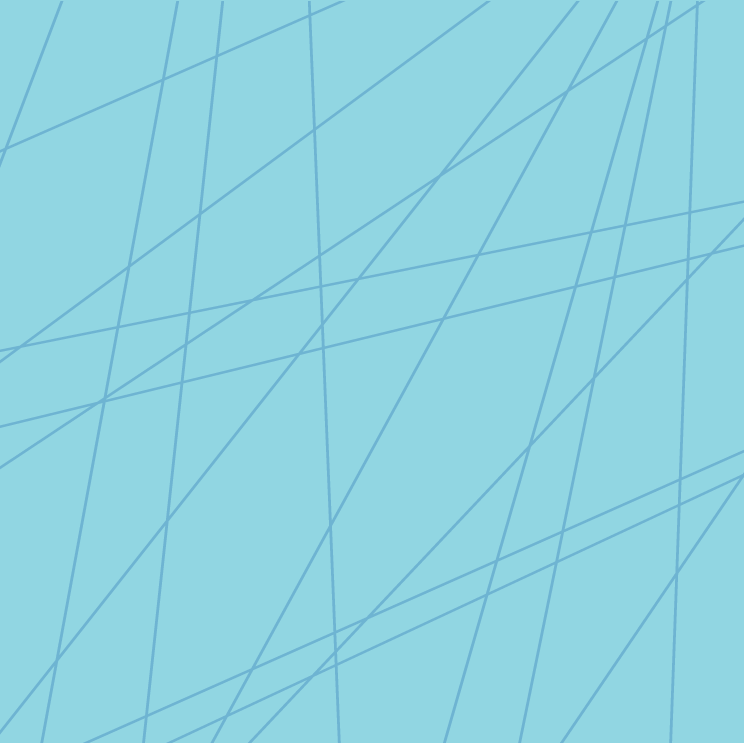
A complementary effort in AI-driven disease prediction and drug discovery is taking place in the lab of Trey Ideker, PhD, professor of medicine, computer science and bioengineering at UC San Diego.
Ideker's team collects genomic, proteomic and cellular imaging data to study diseases such as cancer and autism spectrum disorder and then analyzes the data with machine learning algorithms.
“If we want to understand what drugs work best on different kinds of tumors, for example, we can set up a thousand different tumor cell lines with different gene mutations and expose each to a thousand different drugs,” Ideker said. “Suddenly, we have a billion data points to train an algorithm on instead of waiting on trial and error in human patients to figure out what works or doesn’t.”
This approach is useful for identifying putative drugs and also in refining their clinical trials.
“A clinical trial is much more likely to succeed if it’s focused on people who have the genetic background or are at the stage of disease that the drug is most likely to benefit, as opposed to trying it on a broader population and having no idea why it worked on some people and didn’t work on others,” Ideker said. “Using AI to guide clinical trials allows the people who could benefit from the drug to get it sooner.”
Like Ghosh, Ideker envisions a future in which AI tools are a critical component of the health care process.
“The same way your Netflix algorithm predicts what movie you should watch tonight, our algorithms will predict what drug is best fit to treat your cancer,” he said.
So what’s holding physicians back from using AI in this way now?
According to Ideker, one of the biggest issues is interpretability. Most AI algorithms currently function as “black boxes,” meaning that when they reach a conclusion about something, no one knows exactly how or why that decision was made. This makes it difficult for doctors and patients to trust the recommendations.
“The same way your Netflix algorithm predicts what movie you should watch tonight, our algorithms will predict what drug is best fit to treat your cancer.”
“It’s not enough for an algorithm to just take a complex set of mutations and decide what drug to give a patient if we don’t know why it’s making that choice,” said Ideker. “We may now have enough human genomes sequenced to power precision medicine, but what we don’t have yet is a clear map of cellular biology to interpret the data with.”
To address this, Ideker is leading a series of projects aimed at mapping the structure and function of various cell types, and using these models to inform the AI algorithms. Rather than remaining a black box, Ideker’s team constructs “visible” AI systems in which layers of the neural network are coupled to a hierarchy of cellular processes. The resulting algorithms not only report a drug recommendation but also allow physicians to see which molecular and cellular pathways informed that decision.
This approach recently received support from the National Institutes of Health (NIH) Bridge to Artificial Intelligence (Bridge2AI) program, for which Ideker serves as one of several leading principal investigators. Bridge2AI is the NIH’s largest and most direct investment in AI technology, aimed at advancing its widespread use in research and medicine. Ideker will lead one of four core projects designed to generate comprehensive AI-ready datasets that will lay the groundwork for new, interpretable and trustworthy AI technologies.
“It's not possible to conceive of precision medicine that doesn’t use AI, and it’s a big deal to have NIH recognize that in this way,” Ideker said.
In the new multi-institutional project, Ideker and colleagues will attempt to map the components of a human cell in its entirety, starting with the most basic cell type: the stem cell.
The researchers will obtain induced pluripotent stem cells from a variety of genetic backgrounds and combine microscopy, biochemistry and computational tools to study their biology at multiple scales.
The final product will be a comprehensive model of the architecture of a human cell, from genes and proteins to entire organelles and how they work together. Once the stem cell has been modeled, they plan to use the same approach to model other cells, such as those that are dividing, differentiating or in various disease states.
Their goal is to eventually have a library of cell maps across many demographic and disease contexts, which could be used to train AI algorithms to make informed and interpretable decisions about human health.
“UC San Diego has proven itself to be a leader in clinical and research AI technology, but being part of Bridge2AI is going to be an enormous opportunity for us,” Ideker said.
As he and others continue to lay the groundwork for future advances in biomedical AI, physicians have already begun to embrace their findings in the clinic. Ideker often consults with the tumor board at Moores Cancer Center, where his lab’s research helps guide physicians in designing treatment plans.
“Every cancer patient first tries the established standard of care, but once you’ve gone through those initial options, physicians suddenly have a lot more freedom in what treatment strategies they can recommend,” Ideker said. “While our algorithms aren’t the ones making the decisions yet, it’s exciting to see them finally have a seat at the table.”
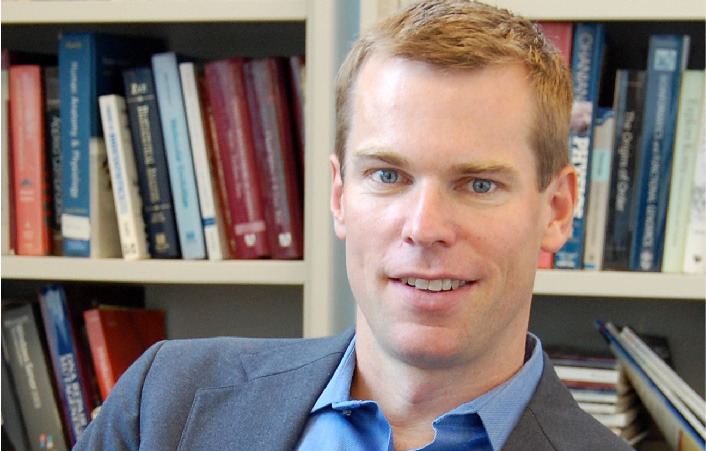
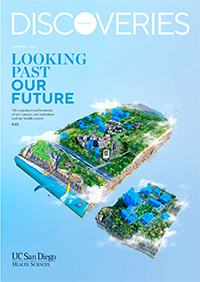
About Discoveries Magazine
This article was originally featured in the 2023 issue of Discoveries, the annual magazine from UC San Diego Health Sciences. Discoveries highlights the latest and greatest in the university's health science research, education and clinical practice. Other stories in this issue explore topics such as mindfulness meditation, wearable medical devices and a profile of new Vice Chancellor for Health Sciences, John M. Carethers.
Share This:
You May Also Like
Stay in the Know
Keep up with all the latest from UC San Diego. Subscribe to the newsletter today.