Making Everyday AI Safe and Trustworthy
Published Date
Article Content
Deep neural networks (DNNs) have transformed industries such as computer vision, healthcare and autonomous driving. However, they remain complex and difficult to understand, and they can fail in unexpected ways, posing significant risks in critical situations. For example, imagine a self-driving car that abruptly stops without explanation, or using an AI-based medical diagnostic tool that delivers inconsistent diagnosis results. Without understanding the reasoning behind these models' decisions, it's hard to trust them when the stakes are high. Additionally, these models often struggle to handle small input changes, compounding the danger in real-world applications.
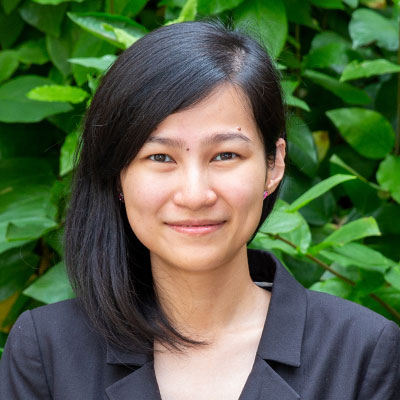
According to Lily Weng, assistant professor at the Halıcıoğlu Data Science Institute (HDSI), part of the School of Computing, Information and Data Sciences (SCIDS) at UC San Diego, current methods for explaining AI decisions focus on highlighting important input features such as pixels in an image, but fail to provide a deeper understanding of how the model processes information.
As a recipient of the highly competitive U.S. National Science Foundation (NSF) Computer and Information Science and Engineering (CISE/IIS) Core Program award, Weng’s project titled “Foundations of Trustworthy Deep Learning: Interpretable Neural Network models with Robustness Guarantees” aims to develop a scalable, automated framework that helps people understand how AI make decisions at the model-level — like a translator that turns complex machine (AI) reasoning into simple, human-friendly explanations, enabling clearer insights into how neural networks think and reason.
“Professor Weng’s research brings analytical rigor and practical systems realization together to advance trustworthy AI that will have a meaningful scientific and societal impact, advancing the mission of the new School on Computing, Information and Data Sciences,” said SCIDS Interim Dean and Founding Director of HDSI Rajesh K. Gupta.
"Receiving this award as a junior faculty and single principal investigator is a meaningful recognition of my lab’s research. It underscores the significance of our work in advancing trustworthy AI and highlights its potential for both scientific and societal impact," Weng said.
Key aspects of the project’s research include leveraging Weng’s expertise in trustworthy machine learning (ML) to develop a scalable, automated interpretation framework. Unlike many existing techniques which are often subjective and difficult to scale, this new framework aims to automatically explain a model’s decision-making process using text-based concepts that are intuitive to humans, similar to the way a teacher breaks down a complex problem into simpler and understandable steps. The framework will also significantly advance the fields of interpretable ML and AI safety by enabling intrinsic, concept-level interpretability within DNNs by design. Ultimately, this project aims to make AI not only smarter but also safer and easier to trust, giving researchers the tools to better understand, control and improve AI systems used in the real world.
With this approach, Weng and her team of graduate students in Trustworthy ML Lab aim to accomplish three objectives: 1) improve transparency, ensuring that AI systems make decisions that can be easily understood and trusted; 2) make debugging simpler by helping researchers and developers quickly identify and fix errors, much like a mechanic diagnosing issues in a car and 3) enable safer AI in high-stakes domains, where timely interventions can be critical.
“By making AI more interpretable and robust, our work will help ensure that deep learning technology is not only powerful but also safe, reliable and widely trusted in everyday life,” said Weng, who also noted the profound societal implications of her team’s project.
“By pioneering more interpretable and robust deep neural networks, we address the urgent need for transparent and reliable AI in critical areas such as healthcare, transportation and criminal justice,” Weng said. “For instance, in healthcare, interpretable models can enhance diagnostic tools, enabling medical professionals to make informed and trustworthy decisions that improve patient outcomes. In autonomous driving, strengthening the robustness and interpretability of AI will contribute to safer navigation technologies, reducing the risk of accidents caused by AI errors. This project represents a critical step toward realizing the promise of AI for the betterment of society.”
Weng shared her excitement about receiving this grant. “It presents the opportunity to push the boundaries of trustworthy AI research and make a tangible impact on both the scientific community and society. I am also particularly excited about the potential to bridge the gap between AI’s impressive capabilities and its trustworthiness. By developing scalable, human-understandable interpretation frameworks, we can move beyond the ‘black box’ nature of deep learning and create AI systems that are not only powerful but also transparent, controllable and aligned with human values,” she said.
Finally, Weng noted that the grant enables her to mentor students and researchers who are passionate about AI safety, fostering the next generation of scientists dedicated to building responsible and ethical AI.
“The prospect of contributing to a future where AI is not only innovative but also safe and trustworthy is truly inspiring,” she said.
This project is supported by the NSF (award no. 2430539).
Learn more about research and education at UC San Diego in: Artificial Intelligence
Share This:
You May Also Like
Stay in the Know
Keep up with all the latest from UC San Diego. Subscribe to the newsletter today.