KnuEdge, UC San Diego Host Conference to Drive Next-Gen Machine Learning
Heterogeneous Neural Networks Conference and Competition in spring 2017 at UC San Diego
Published Date
By:
- Doug Ramsey
Share This:
Article Content
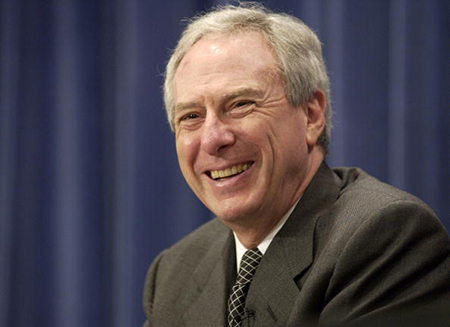
Former NASA Administrator Dan Goldin is CEO of KnuEdge and leading the charge to speed up machine learning via heterogeneous neural networking.
In partnership with the California Institute for Telecommunications and Information Technology (Calit2) at UC San Diego, KnuEdge™ announced the Heterogeneous Neural Networks (HNN) Conference, to be held in spring 2017 in San Diego, Calif. KnuEdge’s LambdaFabric neural computing technology accelerates machine learning and signal processing, The event will also include a KnuEdge-sponsored research paper competition, challenging participants to enable the next-generation of machine learning performance and efficiency through developing heterogeneous neural network algorithms.
"Machine learning has captured the tech industry's attention due to its potential to positively disrupt computing, accelerating cutting-edge technologies ranging from medical research to financial and insurance risk analysis, facial and voice recognition and augmented reality," said Dan Goldin, CEO of KnuEdge. "But to get there, we must encourage and accelerate innovation and unlock what's next in the field. That is why we've decided to launch the Heterogeneous Neural Networking Conference with Calit2."
KnuEdge and Calit2 have worked together since the early days of the KnuEdge LambdaFabric processor when key personnel and technology from UC San Diego provided the genesis for the first processor design. The HNN Conference brings the two organizations back together to focus on advancing machine learning capability and performance.
Preceding the conference, KnuEdge will sponsor a research paper competition for the most innovative and effective heterogeneous algorithms built on today's advanced computing architectures. Currently, there is relatively little opportunity for researchers in the emerging field of heterogeneous neural networks to share their work and collaborate with other experts. This workshop will enable these specialists to learn and exchange ideas with their peers, showcase their work, and gain recognition as pioneers of the discipline.
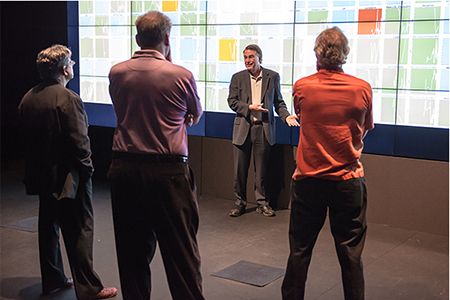
Calit2 Director Larry Smarr thinks neural computing can “deliver on the promise of machine learning.”
Sparse matrix algorithms
The vast majority of machine learning computing today is based on homogenous and convolutional neural network technology, an approach that took hold largely due to the availability of traditional computer architectures, but at the expense of extraordinary computational time and power. Industry thought leaders have long suspected that much greater efficiency and performance could be driven through the use of sparse matrix versus dense matrix multiplication.
For instance, the GoogLeNet paper, “Going Deeper with Convolutions” by Christian Szegedy et al., proposes a solution to overfitting and wasted computation with required large computing infrastructure: "The fundamental way of solving both issues would be by ultimately moving from fully connected to sparsely connected architectures, even inside the convolutions. Besides mimicking biological systems, this would also have the advantage of firmer theoretical underpinnings due to the groundbreaking work of Arora et al... On the downside, today's computing infrastructures are very inefficient when it comes to numerical calculation on non-uniform sparse data structures."
Another paper, “Sparse Convolutional Neural Networks” by Baoyuan Liu et al., argues that “expressing the filtering steps in a convolutional neural network using sparse decomposition can dramatically cut down the cost of computation, while maintaining the accuracy of the system... In our Sparse Convolutional Neural Networks (SCNN) model, each sparse convolutional layer can be performed with a few convolution kernels followed by a sparse matrix multiplication."
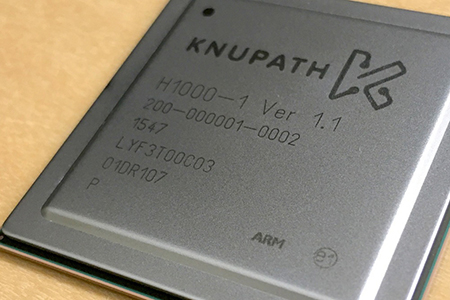
KNUPATH, a unit of KnuEdge, incorporates new neural computing processing technology.
"KnuEdge built its LambdaFabric processor technology to help deliver on the promise of machine learning," said Calit2 Director Larry Smarr, who is also a professor of Computer Science and Engineering in UC San Diego’s Jacobs School of Engineering. "Heterogeneous neural network algorithms are tailored to emulate the efficiency of specific neurobiological pathways that have evolved through natural history. However, this requires the ability to perform sparse matrix multiplication, and hardware optimized for sparse matrix multiplication has been largely unavailable. KnuEdge provides this optimized hardware, enabling analysis of the data as it's delivered from the real world -- allowing much faster computation and time-to-solution."
In October 2015, at Mark Anderson's Future in Review conference, Calit2 Director Smarr announced the formation of a Pattern Recognition Laboratory (PRL), housed in Calit2's Qualcomm Institute. The PRL is dedicated to exploring acceleration of a wide range of machine learning algorithms on novel, non-von Neumann computer architectures. KnuEdge will provide its LambdaFabric technology this year to Calit2's PRL.
"The mission of our Pattern Recognition Lab is to find major increases in energy efficiency and speedups by optimizing machine learning algorithms on novel computing architectures," said PRL Director Ken Kreutz-Delgado, a professor of Electrical and Computer Engineering in the Jacobs School. "We believe this KnuEdge-sponsored contest and conference will accelerate this mission, and we look forward to participating."
Details on the competition, conference agenda, speakers and venue will be released in September 2016. To sign up for email notifications, please contact info@knuedge.com, with the subject line "HNN Conference 2016."
Share This:
You May Also Like
Stay in the Know
Keep up with all the latest from UC San Diego. Subscribe to the newsletter today.