Breakthrough with Quantum Materials Pushes Biologically Inspired Learning Devices
UC San Diego physicists create new nano-scale ‘neuristor’ that mimics brain functions
Published Date
By:
- Mario Aguilera
Share This:
Article Content
To Oleg Shpyrko, the brain is the ultimate device. For certain tasks, the human brain can outperform powerful computers, yet requires the energy output of a light bulb. As one example, the cybersecurity tests required before entering certain websites—picking out pictures of buses and street signs—demonstrate the agility of the human mind over the processing mechanics of a robot.
Several groups of physicists at the University of California San Diego have now taken a leap forward in creating new artificial devices that mimic basic learning functions carried out by neurons in the human brain.
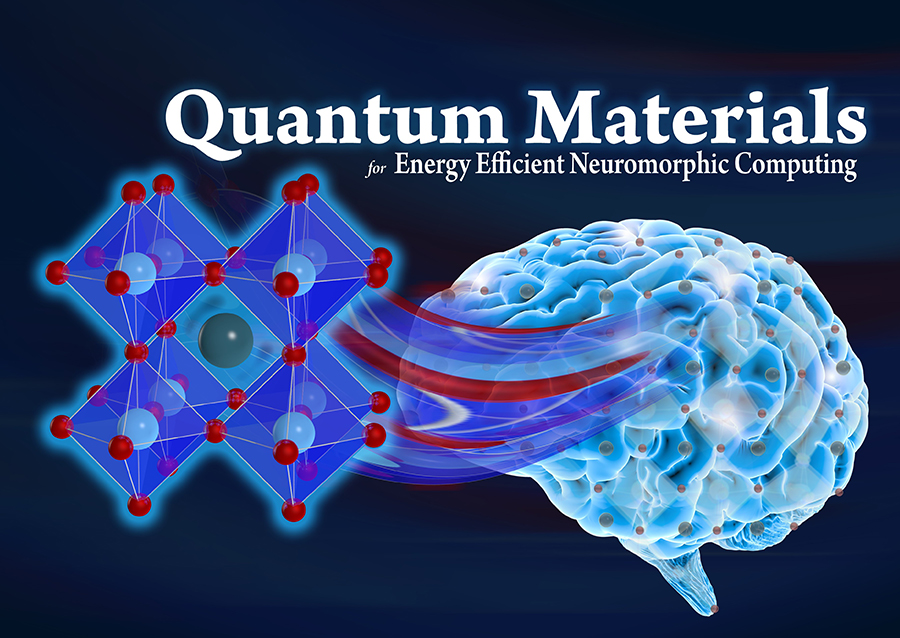
Researchers with UC San Diego’s new Quantum Materials for Energy Efficient Neuromorphic Computing (Q-MEEN-C), a Department of Energy-supported Energy Frontier Research Center, demonstrated how quantum materials—cutting edge substances that are allowing scientists to create new technologies—could be used to develop new devices that can “learn” to switch between functional states.
“The fact that we can make devices that function and are similar to neurons—but perhaps can even be better than the neurons that nature created—is quite surprising,” said Shpyrko. “There are a whole class of tasks where our brains still outperform the traditional semiconductor approaches to computing. We use these brain-inspired approaches to come up with devices that can perform similar types of computation.”
In their efforts to advance biologically inspired brain or “neuromorphic” computing systems, the researchers developed a device made of quantum material that could functionally learn with repeated stimuli. Blending the terms neurons and transistors, these devices are called “neuristors.” With repeated practice inputs, neuristors can learn in the same way that humans figure out how to ride bikes, learn a new language or play the piano. As additional stimuli are reinforced with a skill or task, the more our brains learn.
The researchers published details of their achievement last fall in the journal Small. The key challenge to developing such brain-like devices is to first develop artificial neurons, the cells that send and receive information across the brain and nervous system. Creating neuristors to work like neurons requires control of the nanoscale mechanisms of two basic types of quantum material functions known as volatile and persistent resistive switching.
Neuristors allowed the researchers to control this switching process, opening the door to neuron-like learning via repeated stimuli with minimal energy requirement.
“Improving their energy efficiency makes these neuristors approach the functionalities of neurons in the brain,” says UC San Diego Physics Professor Ivan Schuller, the director of Q-MEEN-C.
“The importance is that for the first time we can take this neuristor device, which simulates behavior that we find in neurons, and we can train it,” said Shpyrko. “Through repeated stimuli the neuristor ‘reprograms’ itself to behave in a very different way than it was functioning before, establishing a persistent conductive pathway. This is an important step towards low-power approaches to neuromorphic computing.”
Having proven the design of the single neuristor, the researchers now plan to build multiple devices that can talk to each other, eventually ramping up to a neuristor network.
“Sometimes a group of people thinking together can be much more powerful than the sum of its parts, somewhat like brain storming,” said Shpyrko. “By allowing the neurons to talk to each other we can create a new phenomenon that could not be explained by a single device but really a network of devices.”
Coauthors of the Small paper include: Anatoly Shabalin, Javier del Valle, Nelson Hua, Mathew Cherukara, Martin Holt, Ivan Schuller and Oleg Shpyrko.
The research was funded as part of the Quantum Materials for Energy Efficient Neuromorphic Computing (Q-MEEN-C) Energy Frontier Research Center (EFRC), funded by the U.S. Department of Energy, Office of Science, Basic Energy Sciences (DE-SC0019273).
Share This:
You May Also Like
Stay in the Know
Keep up with all the latest from UC San Diego. Subscribe to the newsletter today.