By:
- Ioana Patringenaru
Published Date
By:
- Ioana Patringenaru
Share This:
UC San Diego COVID-19 Forecast Now Part of CDC Model
A computational model that forecasts the number of COVID-19 deaths in the United States as a whole and in each state developed by a team of researchers from UC San Diego and Northeastern University is now part of the national mortality forecast issued by the Centers for Disease Control.
UC San Diego joins a roster of prestigious institutions who are included in the CDC’s prediction algorithms, including Harvard, Johns Hopkins and Notre Dame. Among the University of California, three institutions are part of the forecast: UC San Diego, UCLA and UC Merced.
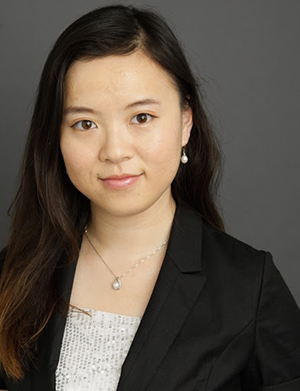
Rose Yu, an assistant professor in the Department of Computer Science and Engineering, is one of the two researchers leading the UC San Diego modeling work.
“Our goal is to provide insights to policymakers as they make decisions about reopening,” said Yian Ma, an assistant professor at the UC San Diego Halicioglu Data Science Institute who co-leads the UC San Diego modeling effort with Rose Yu, assistant professor in the Department of Computer Science and Engineering.
Currently, the model is predicting a steady increase in the number of deaths during flu season, without dramatic spikes.
Ma and Yu are partnering with Matteo Chinazzi and Alessandro Vespignani at the Network Science Institute at Northeastern University, a research group that regularly consults about the pandemic for the CDC.
The UC San Diego-Northeastern model, called DeepGLEAM, is unique because it combines a physics-based model, known as GLEAM, with deep learning—a computing system made up of algorithms inspired by the way the human brain is organized. The hybrid model leverages rich data information about COVID-19 from the real world, such as when a person had been infected and where they have traveled.
“Combining the two is important,” said Yu. The physics-based model is not good at handling uncertainty and unknowns in the data, for example how well people adhere to travel bans. That’s where deep learning comes in to help reduce uncertainty.
Other research teams might have expertise in one area or the other. The UC San Diego-Northeastern team has experts in both.
The experts
Yu is an expert in the nuts and bolts of deep learning and spatiotemporal modeling. She was an assistant professor at Northeastern University before joining UC San Diego. While at Northeastern, she worked with Vespignani. “She was our resident expert on machine learning and neural networks,” he said. “We thought she would be the perfect partner for this project.”
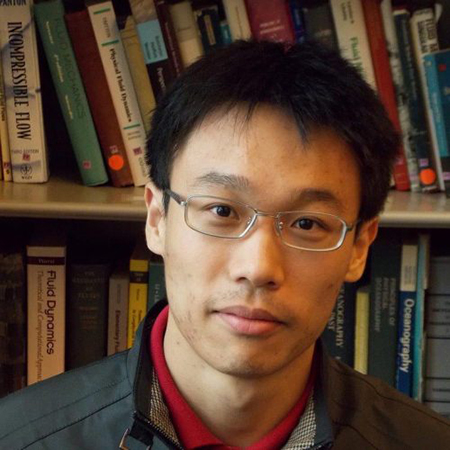
Yian Ma, an assistant professor at the Halicioglu Data Science Institute, co-leads the modeling effort at UC San Diego.
Ma is also a machine learning specialist, although his focus is on theory. He started working on the project right after a stint as a visiting scientist at Google Research. He and Yu asked Vespignani if it still made sense to work on a long-term forecasting model. Vespignani said yes. “So we thought: ‘We should get to work,’ ” Ma said.
Vespignani is a well known scientist who leads the Network Science Institute at Northeastern. He and his team develop analytical and computational models for large-scale social, technological and biological networks. This allows them to model contagion and predict the spread of emerging diseases. He was profiled in The New York Times in March of this year for his work on predicting the spread of the coronavirus.
The model
The model Ma, Vespignani and Yu developed is based on predictions from a physics-based model using a wealth of data: death certificates, information about when the deceased contracted COVID-19 and their previous movements as well as information about the various travel and opening restrictions from all 50 states. But based on this information, there is still a lot the researchers don’t know: for example, how well people adhere to restrictions.
That’s where machine learning comes in. The algorithms and computational networks are able to handle uncertainty and make predictions. It’s tricky, Ma said, because researchers have to make sure they create their system to provide accurate and meaningful results while accounting for uncertainty. “We want to get it right,” he said.
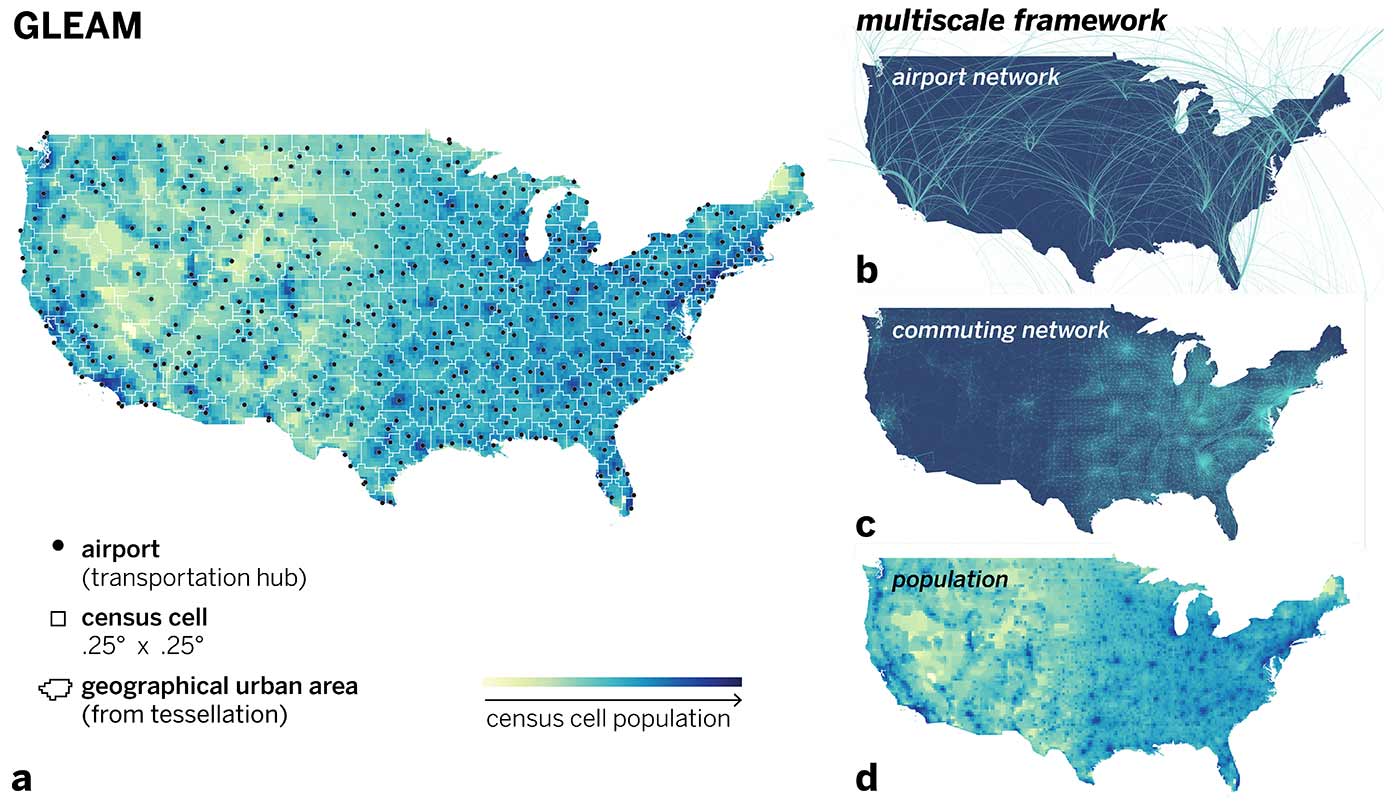
A schematic representation of the Global Epidemic and Mobility (GLEAM) model for the United States. The country is divided into census cells that are assigned to subpopulations centered around transportation hubs. The population layer describing the census cells is coupled with two mobility layers, the short-range commuting layer and the long-range air travel layer.
For now, the model is most accurate one week out, and successively less so for two, three and four weeks out.
“We want to make weekly forecasts, which can be useful to policymakers,” Vespignani said.
The CDC does use the data, which are available publicly, in a timely manner. But on average, the information you’d see today is a week old.
The researchers’ next steps include creating forecasts for all counties in the nation, updated once a week.
“And, of course, we are always updating our model on a weekly basis to make it as accurate as possible,” Yu said.
Share This:
Stay in the Know
Keep up with all the latest from UC San Diego. Subscribe to the newsletter today.