Nanopore Technology Makes Leap from DNA Sequencing to Identifying Proteins
Published Date
By:
- Doug Ramsey
Share This:
Article Content
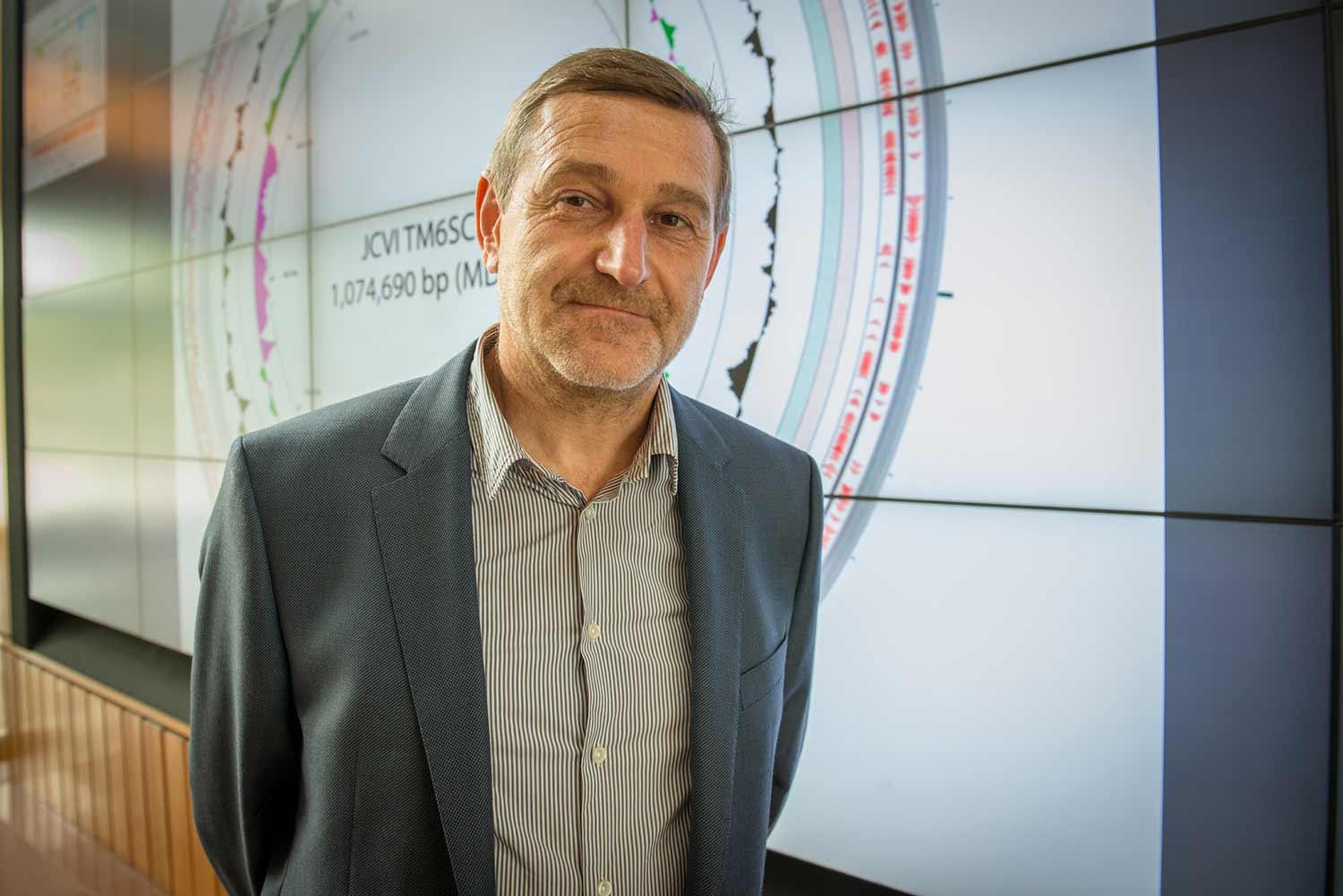
UC San Diego computer scientist Pavel Pevzner, senior author on the new study in PLOS Computational Biology
In the May issue of PLOS Computational Biology, scientists from UC San Diego and the University of Notre Dame report on a study that could open up the field for nanopore-based protein identification – and eventually proteomic profiling of large numbers of proteins in complex mixtures of different types of molecules.
According to UC San Diego computer science and engineering professor Pavel Pevzner, senior author on the paper, the new approach identifies proteins by analyzing the distinct electrical signals produced when the molecules pass through a nanopore (which acts like a sieve). In theory, says Pevzner, nanopores could allow researchers to characterize large numbers of proteins in complex mixtures.
While nanopores work extremely well in analyzing single molecules, they are less effective when trying to characterize large numbers of proteins in complex mixtures. As a result, the currently preferred approach to screening complex mixtures involves using other techniques, notably mass spectrometry. (Pevzner and CSE professors Vineet Bafna and Nuno Bandeira are principal investigators of the NIH-funded Center for Computational Mass Spectrometry at UC San Diego.)
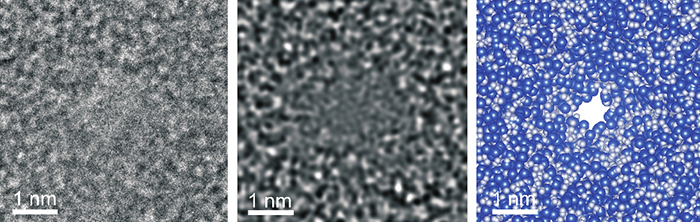
Transmission electron microscopy (TEM) micrograph of sub-nanopore; at right, silicon atoms represented by spheres 0.235 nanometers in diameter, while nitrogen atoms are sphere 0.13nm in diameter.
As recently as 2016, leading nanopore developers were pessimistic about being able to apply nanopores to large-scale protein profiling in the near term. “We aren’t even close to doing that at the moment,” Oxford Nanopore co-founder Hagan Bayley told GenomeWeb, adding that he “wouldn’t say it’s an impossible goal, but it is a bit of a stretch.”
UC San Diego’s Pevzner, however, believes that a breakthrough is at hand. “The key is to use machine learning to analyze information generated by proteins when they translocate through a nanopore,” said Pevzner. “By applying machine learning techniques, we were able to identify distinct signals that could lead to large-scale nanopore protein analysis.”
In an interview with GenomeWeb, Pevzner says that, early on, the obstacles appeared intractable. "The data was so noisy that we almost thought we should give up," he explained. "I have been working for almost 10 years now on top-down mass spectrometry, and in comparison with protein identification by top-down mass spectrometry, which by now is almost a mature area, it looked like there was no hope that nanopores could produce a comparable signal."
Then, when the researchers applied a random forest analysis tool from machine learning to the problem, everything changed. Recalled Mikhail Kolmogorov, a graduate student in Pevzner’s lab: “All of a sudden, the structure of the signal emerged.”
As stated in the PLOS paper, the researchers argue that “the current technology is already sufficient for matching nanospectra against small protein databases, e.g., protein identification in bacterial proteomes.”
*Kolmogorov M, Kennedy E, Dong Z, Timp G, Pevzner PA. “Single-molecule protein identification by sub-nanopore sensors,” PLOS Computational Biology, 13(5): e1005356, May 2017.
Share This:
You May Also Like
Stay in the Know
Keep up with all the latest from UC San Diego. Subscribe to the newsletter today.