Kamalika Chaudhuri: Quantifying the Price of Privacy
By:
- Tiffany Fox
Published Date
By:
- Tiffany Fox
Share This:
Article Content
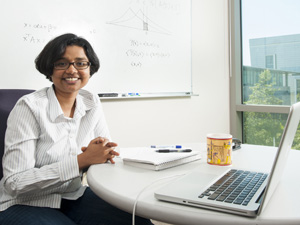
Kamalika Chaudhuri
The data avalanche brought about by the digital revolution has made it possible to harness vast datasets for everything from statistical analysis to teaching machines to recognize patterns and respond in ‘intelligent’ ways.
But much of this data comes from humans, and many of those humans expect their data to remain private. Preserving this privacy, however, is not always easy, says University of California, San Diego Computer Science Professor Kamalika Chaudhuri.
“Suppose you have some sensitive data, such as genomic data that you’ve gathered from patients, and now you want to compute some statistics on that data to develop some kind of prediction algorithm,” she explains. “For example, you could be analyzing certain features of patients in order to predict if they might develop a certain disease.
“With most data-based research, so long as the patients’ names and addresses and some other identifying information are removed, the data is considered private,” adds Chaudhuri, an affiliate of the UC San Diego division of the California Institute for Telecommunications and Information Technology (Calit2), where she worked as a researcher for Calit2’s Information Theory and Applications Center.
“But with datasets with a lot of features, this is not the case,” she notes, “particularly when they are based on small sample-sizes.”
Privacy researchers have discovered that with a little prior knowledge it is possible to ‘reverse-engineer’ the statistics obtained from such data to determine who the patients are, thus compromising their privacy.
To account for this, Chaudhuri and her team have developed a series of privacy-preserving techniques — collectively known as “Differentially Private Empirical Risk Minimization” — to determine how to classify data and subsequently develop prediction algorithms, while simultaneously maintaining privacy.
Chaudhuri will present a paper on her approach, titled Convergence Rates for Differentially Private Statistical Estimation, at the upcoming International Conference on Machine Learning June 26-July 1 in Edinburgh, Scotland.
One crucial aspect of the approach is to add a certain degree of noise to a dataset to mask the effects of one person’s data being added (aka “objective perturbation”).
“But because you’re adding a little bit of noise to the data, you’re also losing some accuracy,” notes Chaudhuri, “so we also try to quantify how much accuracy you’re going to lose in order to provide privacy. The more samples in your data, the less the relative loss of accuracy, so accuracy is a function of sample size. So really what we’re doing is quantifying the price of privacy.”
Chaudhuri says her team’s results show that, both theoretically and empirically, objective perturbation is superior to previous state-of-the-art techniques for managing the inherent tradeoff between privacy and learning performance. Better yet, the techniques can be used for any type of data — from medical data to financial data — thereby ensuring that machines can get smarter without compromising the human desire to remain anonymous.
Chaudhuri’s newest paper on the topic, which she co-authored with UC San Diego postdoctoral researcher Daniel Hsu, investigates the kind of properties statistical estimators should have so that they can be computed with differential privacy and relatively low loss in accuracy. The results in the paper reveal a concrete connection between differential privacy and robust statistics, a field of statistics that builds statistical estimators which account for small errors and outliers in the data.
“Much of data-based research involves the computation of some kind of statistical estimator, such as the mean, the median or p-values, based on the data,” Chaudhuri explains. “When this data is sensitive, these estimators need to be computed with privacy. Our results indicate that statistical estimators, which are robust in a certain sense, can be approximated with differential privacy and high accuracy."
Share This:
You May Also Like
Stay in the Know
Keep up with all the latest from UC San Diego. Subscribe to the newsletter today.