Conference on Mathematical Theory of Deep Neural Networks (DNN) at Halıcıoğlu Data Science Institute
Story by:
Published Date
Article Content
On Nov 17-18, 2022, held its first in-person DeepMath conference on campus since the pandemic, which included several HDSI faculty, postdocs and graduate students. This was the fifth iteration of the conference, which had been hosted virtually during the previous two years.
Many recent advances in DNNs, combined with open, easily-accessible implementations, have made DNNs a powerful, versatile method used widely in machine learning, with applications across many domains, including neuroscience. These advances in practical results, however, have far outpaced a formal understanding of these networks and their training. The dearth of rigorous analysis for these techniques limits their usefulness in addressing scientific questions and, more broadly, hinders systematic design of the next generation of networks. Recently, long-past-due theoretical results have begun to emerge from researchers in a number of fields shedding light on understanding and application.
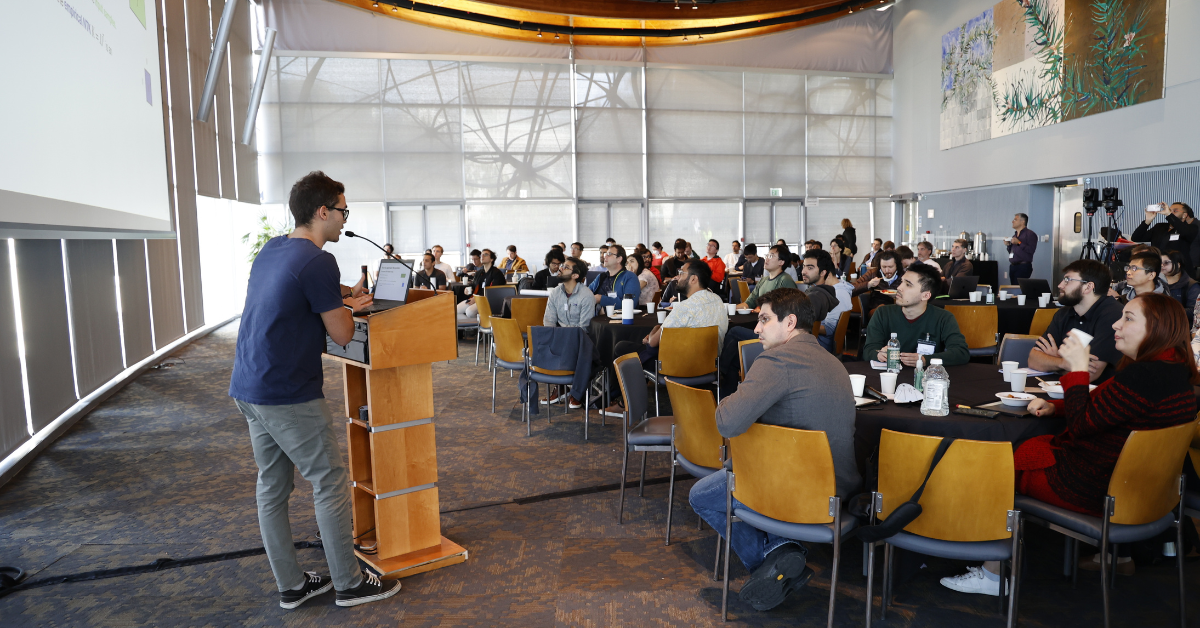
The purpose of DeepMath this year was to give visibility to recent advances in deep neural networks (DNNs), and those that will follow in their wake, to shed light on the properties of large, adaptive, distributed learning architectures, and to revolutionize our understanding of these systems.
DeepMath is committed to fostering dialogue between people working on the technical development of these technologies and those with a broader view of how they are being implemented.
The two-day conference, brought together students from and hosted seven invited speakers from various institutions in the data science world, both domestic and international. There were two full days of activities, to engage participants, including an enthusiastic poster session on Thursday evening.
This year's sponsors of the event were the National Science Foundation (NSF), Simons Foundation, John Hopkins Mathematical Institute of Data Science and HDSI. DeepMath is committed to diversity and for the first time offered travel grants designed to reduce barriers to participation and broaden representation in the field of the mathematical theory of deep neural networks. This year funding was provided to multiple individuals to cover the cost of care for dependents during the conference, and this effort will be expanded to additional types of grants to support participation in upcoming years.
As part of that effort, this year one of the invited speakers was Berk Ustun, an assistant professor at HDSI. Berk says, "We can now build deep learning models that support important decisions or automate routine tasks. Yet we may not reap their benefits due to disuse, or inflict harm due to misuse. Some of the best researchers who are best-equipped to discuss these issues work in other areas of machine learning, or even other disciplines like Medicine and Law. Creating room for this dialogue can help draw attention to new problems and ultimately develop more reliable technical solutions."
“We can now build deep learning models that support important decisions or automate routine tasks. Yet we may not reap their benefits due to disuse, or inflict harm due to misuse. Some of the best researchers who are best-equipped to discuss these issues work in other areas of machine learning, or even other disciplines like Medicine and Law. Creating room for this dialogue can help draw attention to new problems and ultimately develop more reliable technical solutions."
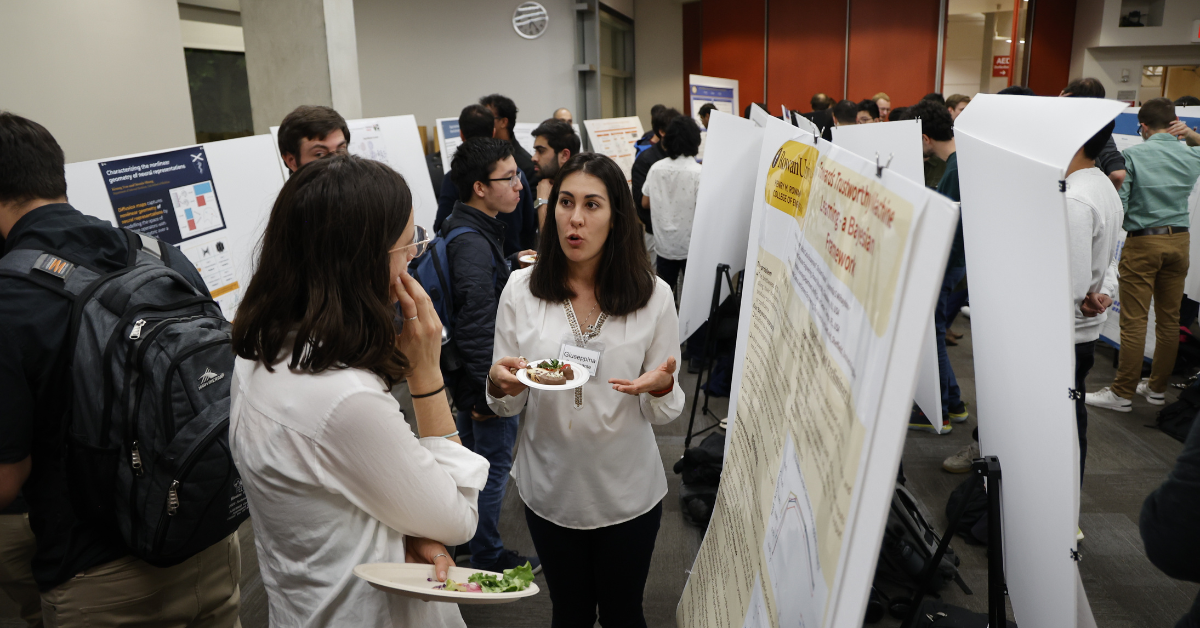
The next annual Deep Math Conference is scheduled for November 2023.
For questions regarding this article and other HDSI information, please contact HDSIComm@ucsd.edu.
Share This:
You May Also Like
Stay in the Know
Keep up with all the latest from UC San Diego. Subscribe to the newsletter today.